Hydro Thunder(Arcade) The Far East 1:19.46
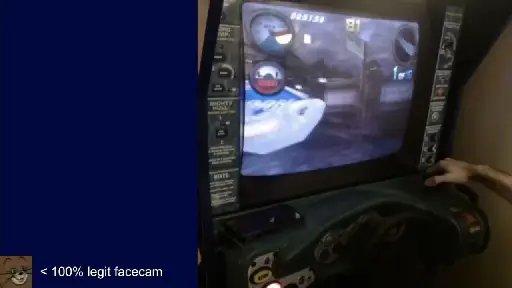
YouTube Video
Click to view this content.
Super Mario Odyssey Any% (2 Player) in 56:54
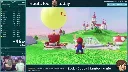
zackintheboxx went live on Twitch. Catch up on their Super Mario Odyssey VOD now.
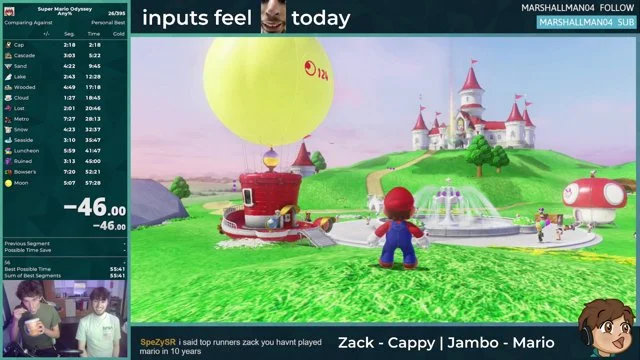

Highlights - Cycle Ball - Gold Medal Final | 2021 UCI Indoor Cycling World Championships

YouTube Video
Click to view this content.

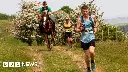
The winner outran the fastest horse by over 10 minutes in the 22-mile race held in mid Wales.
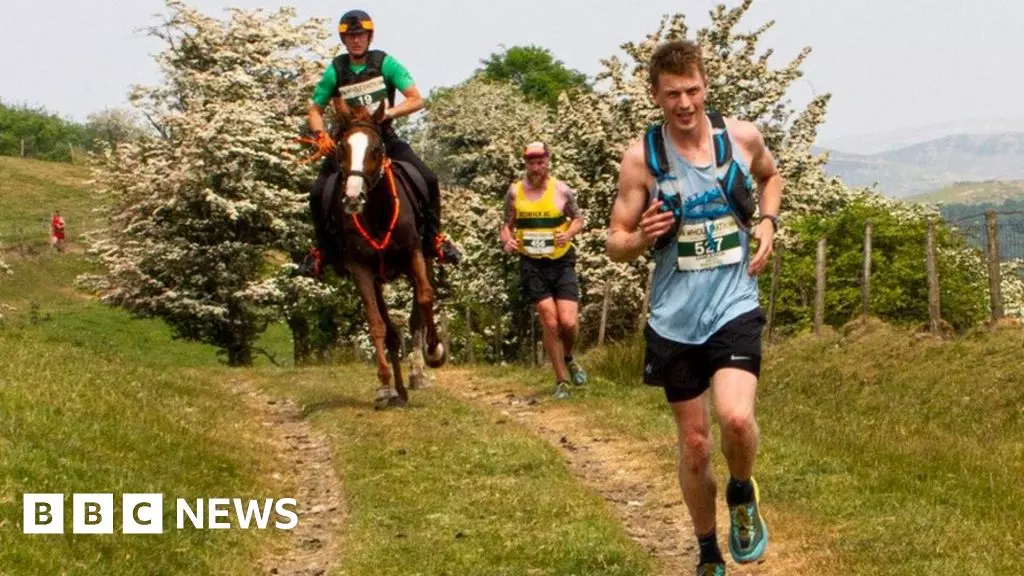

Milwaukee Senior TT - Highlights | 2023 Isle of Man TT Races

YouTube Video
Click to view this content.

open source math textbooks

A curated list of awesome mathematics resources. Contribute to rossant/awesome-math development by creating an account on GitHub.

some links are broken but otherwise good. Post your open source math textbooks here

After state board approves first taxpayer-funded Catholic school, Hindus seek same | KGOU
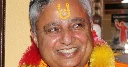
As Oklahoma pushes ahead with plans for the first ever taxpayer-funded Catholic public charter school, some say other religions should be included.
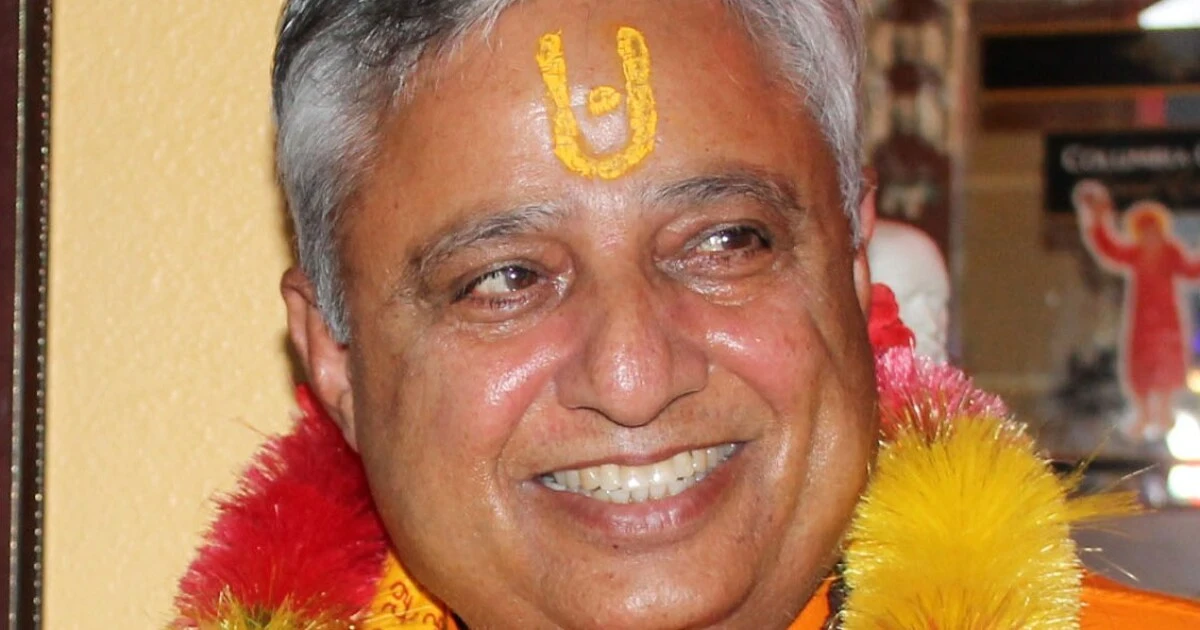
cross-posted from: https://lemmy.sdf.org/post/43170

"Prompt Gisting:" Train two models such that given inputs "Translate French
<G1>
<G2>
" and "<G1>
G2>The cat," then G1 and G2 represent the entire instruction.cross-posted from: https://lemmy.sdf.org/post/36227
Abstract: "Prompting is now the primary way to utilize the multitask capabilities of language models (LMs), but prompts occupy valuable space in the input context window, and re-encoding the same prompt is computationally inefficient. Finetuning and distillation methods allow for specialization of LMs without prompting, but require retraining the model for each task. To avoid this trade-off entirely, we present gisting, which trains an LM to compress prompts into smaller sets of "gist" tokens which can be reused for compute efficiency. Gist models can be easily trained as part of instruction finetuning via a restricted attention mask that encourages prompt compression. On decoder (LLaMA-7B) and encoder-decoder (FLAN-T5-XXL) LMs, gisting enables up to 26x compression of prompts, resulting in up to 40% FLOPs reductions, 4.2% wall time speedups, storage savings, and minimal loss in output quality. "

Taming AI Bots: Prevent LLMs from entering "bad" states using continuous guidance from the LLM ("is this good? bad?") to avoid bad states.

The space of homogeneous probability measures on $\overline{\Gamma \backslash X}_{\max}^{S}$ is compact